Biomarker discovery
A biomarker is typically defined as a characteristic that is objectively measured and evaluated as an indicator of normal biological processes, pathogenic processes, or pharmacological responses to a therapeutic intervention.
The term biomarker discovery is inextricably linked with modern pharmaceutical and biotechnological research. In general this term means after measuring the biological raw material the whole workflow from data preprocessing up to the reduction of high-dimensional genomics data resulting in diagnostically conclusive data subunits. The applications vary from, e.g., distinguishing cancer tissues from normal tissues to predicting different disease states and the diagnosis of complex diseases at the molecular level.
Feature selection
Technically speaking the conception behind is to be able to predict distinguishable states (classes) based on measured biological material. Thus, the essential step in biomarker discovery is feature selection: The reliable identification of variables with predictive power.
Feature selection originates in machine learning and statistics where redundant features do not provide additional information to the currently selected features, and irrelevant features provide no useful information in any context. It is obvious that avoiding these feature types decreases the computational costs in later steps, e.g. when training and applying the predictive model, but one might naively expect this to be the only advantage. In fact, feature selection is a central task since it influences the predictive accuracy. The best selection algorithms reduce the risk of overfitting and, hence, improve the accuracy. Finally, regarding the biological background these methods have another merit:
Models based on few biomarkers are often easier to interpret (biologically, e.g. connections between genes).
Scientific Consilience
Scientific Consilience has specialized in analyzing biological data. Biological data have several peculiarities rendering the analysis a challenging task. At its simplest, they are covered with noise and measurement errors. Often, statistical hypotheses fail due to the lack of assumed data distributions - which may be an individual entity of the corresponding measuring device.
TrueSet is Scientific Consilience's proprietary biomarker selection service. We have developed proprietary software as part of our analysis service combining information theoretical criteria with statistical evaluations.
Workflow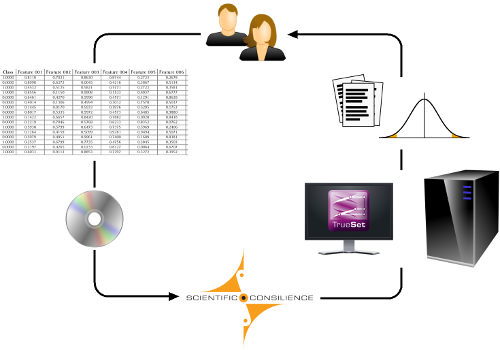
Commonly, our customers provide us with anonymized samples, e.g. vectors of numbers labeled with class identifiers which we analyze and preprocess. Based on these data, we select the best biomarkers, do all statistical tests and predictions in-house, and return the desired results. When performing feature selection, we usually identify the optimal number first and then select the best biomarkers for subsets of a given size. Based on these findings, we train a predictive model, which may be used for predicting the properties or classes of new samples. This model is then transferred to our customer, who may use the model for future evaluations.
Please feel free to contact us for more information under
info (at) scientific-consilience.com